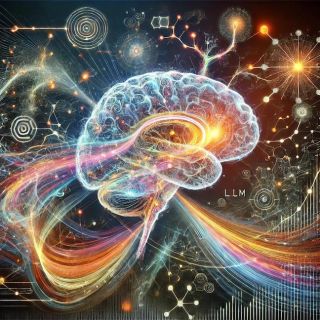
Source: Art: DALL-E/OpenAI
In the complexity of human cognition, the hippocampus stands as a central player, orchestrating more than just the storage of memories. It is a master of inference—a cognitive ability that allows us to go beyond the raw data we receive and extract abstract relationships that help us understand the world in more flexible and adaptable ways. A recent study in Nature underscores this point, showing that the hippocampus encodes abstract, high-level representations that facilitate adaptive behavior and generalization across different contexts.
At its core, inference is the cognitive mechanism by which we draw conclusions from known facts, even when the information is incomplete or ambiguous. This ability is what allows us to understand metaphors, predict outcomes, and solve problems—often with very little data to go on. In the hippocampus, this process relies on the ability to compress information into abstract representations that can be generalized and applied to new situations. Essentially, the hippocampus enables us to think beyond the present, drawing connections and making predictions that guide our decisions and actions.
But what about machines? Can Large Language Models, which operate based on predictive algorithms, emulate this kind of higher-order cognitive function?
LLMs and the Art of Predictive Inference
At first glance, LLMs might seem like simplistic statistical machines. After all, their primary function is to predict the next word in a sequence based on patterns they’ve seen in vast datasets. But beneath this surface lies a more complex mechanism of abstraction and generalization that, in some ways, mirrors the hippocampal process.
LLMs don’t just memorize word pairs or sequences—they learn to encode abstract representations of language. These models are trained on immense amounts of text data, allowing them to infer relationships between words, phrases, and concepts in ways that extend beyond mere surface-level patterns. This is why LLMs can handle diverse contexts, respond to novel prompts, and even generate creative outputs.
In this sense, LLMs are performing a kind of machine inference. They compress linguistic information into abstract representations that allow them to generalize across contexts—similar to how the hippocampus compresses sensory and experiential data into abstract rules or principles that guide human thought.
Bridging the Gap: From Prediction to True Inference
But can LLMs really achieve the same level of inference as the human brain? Here, the gap becomes more apparent. While LLMs are impressive at predicting the next word in a sequence and generating text that often appears to be the product of thoughtful inference, their ability to truly understand or infer abstract concepts is still limited. LLMs operate on correlations and patterns rather than understanding the underlying causality or relational depth that drives human inference.
In human cognition, the hippocampus not only predicts what’s likely to come next based on past experience but also draws from a rich understanding of abstract relationships between objects, ideas, and experiences. This allows humans to make leaps in logic, solve novel problems, and apply learned principles across vastly different scenarios.
To push LLMs toward a more advanced level of inference, we would need to develop systems that move beyond merely predicting the next word based on statistical probabilities. We would need to design models that can encode abstract principles and relationships in a way that allows them to apply these ideas flexibly across different contexts—essentially creating a kind of “LLM hippocampal functionality.”
Building the Future of Inference in AI
The idea of developing LLMs with hippocampal-like functionality is tantalizing. Such systems would not just predict the next word but would have a deeper, more abstract understanding of the information they process. This would open the door to machines that could infer complex relationships, draw novel conclusions from minimal data, and apply learned principles across diverse situations—mirroring the flexibility of human cognition.
Several avenues could be explored to move LLMs closer to this goal. One promising direction is the incorporation of multimodal learning, where LLMs would not just process text but integrate information from various sensory inputs, such as images or sounds, to develop a more holistic and abstract understanding of the world. Additionally, advances in reinforcement learning, where models learn through trial and error in dynamic environments, could help simulate the way humans learn and infer from real-world experiences.
Ultimately, the future of AI may lie in creating systems that more closely emulate the abstract, generalizable reasoning that the hippocampus provides for humans. These “next-gen” LLMs would not only predict but also infer, reason, and adapt to new situations with a level of flexibility that currently remains uniquely human.
Let’s Infer the Future
The interplay between human cognition and machine intelligence continues to evolve, and the next leap in AI could very well involve bridging the gap between prediction and inference. By looking to the hippocampus and its role in abstract reasoning, we may uncover new ways to design AI systems that think more like us—not just anticipating the future but also understanding the underlying patterns that make the future possible. The question isn’t just whether LLMs can predict the next word in a sentence but whether they can begin to understand and infer the world in a way that mirrors the richness of human thought. If we can achieve this, the potential for AI to become not just a tool, but a cognitive partner, grows ever closer.